Organizations are drawn to the promise of AIOps to leverage AI-driven Intelligence and automation to make quick and accurate decisions to maintain resiliency. AIOps uses artificial intelligence to simplify IT operations management and accelerate and automate problem resolution in complex modern IT environments.
A recent blog by Sanjay Chandru set the stage for guiding you on Best practices for taking a hybrid approach to AIOps. We learned that a key capability of AIOps is deciding root causes. Accurately diagnosing and deciding how to fix problems quickly in dynamic and complex environments across hybrid cloud infrastructure and applications empowers IBM Z IT ops teams, and accelerates the AIOps journey.
In this blog we will focus on performance and capacity planning. A broad topic encompassing reporting forecasting for improved usage management of resources and planning for tomorrow with advanced modeling.
What are the challenges faced today?
The role of performance management is essential for setting, evaluating and understanding the goals of IT resources and how it relates to the overall need of the business. In an increasingly complex hybrid cloud environment, understanding the relationship between applications and resources is critical to ensure that Service Level Agreements and other performance goals are being met and, when not, the right information is available to appropriate stakeholders to make prompt, accurate decisions on the actions to be taken.
When making capacity planning decisions, the focus is often on making the best use of existing resources and where to make smart investments, for example, purchasing of new hardware or upgrading to latest technologies. Blindly making these capital investments can result in mistakes where the expected benefits are not realized. Pulling together the right data make the correct decision can be difficult and time consuming, to say nothing of the skills of the capacity planner that may be needed to make judgements and recommendations.
In summary, many enterprises struggle today with curating the key data points needed to make business decisions about the ever-changing needs of their IT environments. The inability to do this effectively slows down the decision-making process that increases the time to conclude the most appropriate actions to take and ultimately affects business outcomes.
What’s now required and how different then what I have today?
All enterprises today will have some tooling for performance management and capacity planning, and some license multiple products for similar or overlapping tasks which is of course, inefficient. Many have a reliance on homegrown solutions built by domain specialists over many years. These tools are now often unable to provide the reporting and functionality required, either because the creator of the tooling is no longer around, or that assumptions around how workloads were expected to be used and the impact on performance are no longer valid.
An example of these changing assumptions can be seen around the level of expectation to resolve problems. Traditionally, performance reporting could be satisfied by generating reports the following day after processing operational data such as SMF records overnight. With the mainframe being part of integrated environment, workloads are being driven increasingly as part of hybrid applications using API-enabled resources. Given the volume of SMF data that can be produced, it’s understandable that many look at ways of avoiding processing during peak periods with the result that detailed reporting cannot be made available until the next day.
It is no longer acceptable to wait until the following day to access information to investigate root cause of performance issues or produce data-driven insights to align with other enterprise-wide reports. Additionally, self-service access to reports and information empowers the end user from management to technical stakeholders to access and customize the information to their needs instead of depending on one or two skilled report builders. Untimely access to information for managing workloads and making decisions around resource optimization resources can impact service levels and drive-up operational costs.
The IBM solution and key differentiation
The principal solution to address these challenges is IBM Z Performance and Capacity Analytics.
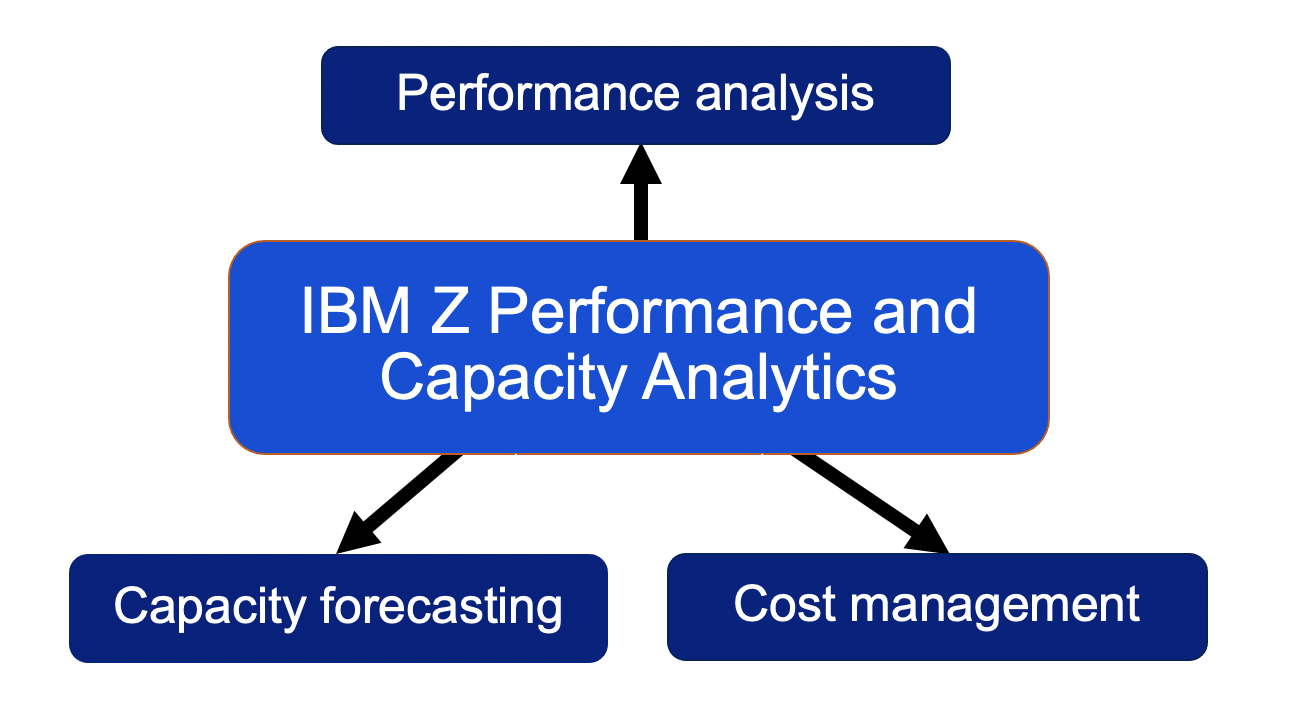
We talk about three key areas of capability:
- Performance Analysis is the task of measuring and checking the level of performance in terms of productivity and usage trends. It involves taking the data about the environment and workloads to draw out important insights about changes in performance to support subject matter experts in their analysis.
- Capacity Forecasting ensures that the capacity of IT infrastructure is able to deliver the agreed service level targets. Now we’re not just using the data for reactive analysis but also for proactive tasks that will help the business make accurate and timely decisions to make the most of existing investments and future needs.
- Cost Management helps you give accurate and cost-effective stewardship of assets and resources used in providing IT services. This includes leveraging the data for chargeback or showback to consumers.
SMF records produce a wealth of data but that can be a challenge for many to turn into insights within a timely fashion and make the correct decision about what to do. What do I need to collect and what to analyze? Large amounts of SMF data can also be costly to process and so missing insights or being too slow to react defeats the point of collecting this data.
The Key Performance Metrics are an out-of-the-box summary of the essential metrics most performance analysts would typically want to use. Many of these reports have been designed in conjunction with subject matter experts in particular domains (for example, z/OS, hardware, storage, CICS or IMS) that delivers immediate value. The processed data can then be quickly used for making forecasts or simulations on potential future performance to support capacity planning activities.
This is also achieved while also lowering overall processing overhead typically seen with SMF data. There are several parts to this, for example the Key Performance Metrics feature reduces the amount of data needed to be collected, together with an architecture that curates data in a continuous near real-time model resulting in low but regular processing versus a large batch process of SMF records. Many components within the product are also zIIP-eligible meaning less impact on general processors.
Simplifying the user experience and delivering real value
In one example, a major US-based bank was looking at modernizing their entire performance management, capacity planning and chargeback processes as their current tooling and organizational structure did not fit with the modern way they needed to support multiple internal customers on the mainframe. Significant periods of time was taken creating and updating reports, making manual changes to work around known problems and prepare chargeback billing. All of this was a drain on the team and prevented them from looking at higher value tasks. Additionally, there was a lack of satisfaction from their internal consumers and customers due to the slow response and lack of transparency.
Working with the client, we assisted in the deployment of IBM Z Performance and Capacity Analytics and creating a new infrastructure based around a hub database of operational data that is leveraged by the team to perform near real-time performance analysis and capacity management on key resources. Next, the data was used as a basis for capacity planning activities allowing them to make accurate forecasts on future consumption. Using a mix of pre-defined and customized reports, the team was able to build a self-service portal for their internal clients to look up their current resource usage on the mainframe at any time. This model enables greater transparency between IT operations team and the lines of business as they are able to show exactly what resources are contributed to the billing. Following a successful deployment, the legacy toolsets were able to be decommissioned reducing the number of tools and licenses to be maintained.
Next steps
Depending on where you are on your journey to adopting AIOps best practices we are sharing the following resources to obtain a deeper understanding:
- To assess your current stage of AIOps maturity and identify action oriented next steps for adopting more AIOps best practices, ask your IBM representative about the 15-minute online AIOps Assessment for IBM Z.
- Learn more about IBM Z Performance and Capacity Analytics and how it can provide insight supporting strategies to optimize workload performance and cost in this webinar on August 19, 2021 (or on-demand after).
- Join the AIOps on IBM Z Community to follow this blog series about best practices for taking a hybrid approach to AIOps and other topics.
Originally published on the AIOps on IBM Z Community Blog