By now, I’m sure you are well aware of the digital transformation that has been going on for some time. As IBM Z continues to be a cornerstone of our customer’s hybrid cloud solutions, the need for resiliency, avoiding operational issues and service degradation has become even more critical. The Journey to AIOps is how IBM is infusing AI and machine learning into our solutions to help our customers become more intelligent in their operations. IBM Z Operations Analytics uses this technology to intelligently detect operational anomalies in both operational log and metric data. By identifying operational anomalies, Z Operations Analytics helps customers proactively avoid costly incidents.
Earlier this month, a continuous delivery PTF was made available for IBM Z Operations Analytics that expanded subsystems supported by delivering enhanced support for CICS. The CICS subsystem support includes more than 70 new KPIs across 5 different KPI groups in addition to the KPIs previously defined for Db2. To build the KPI list, the Z Operations Analytics data science team worked closely with the CICS development organization and CICS user group to understand the most meaningful KPIs and data needs.
The KPI groups that are supported for CICS along with some of the key KPIs are in the list below. The complete list can be found in the solution documentation.
- Communication: Socket Detail such as peak, maximum sockets
- Dispatcher: Tasks, TCB CPU
- Dumps: System dumps, transaction dumps
- Storage: Short on storage
- Tasks: Transaction counts, cpu time
In order to detect operational anomalies, Z Operations Analytics uses IBM Watson Machine Learning for z/OS to build a model of your environment based on normalized historical data for each of the KPIs. Once the model is built, real time KPI data is compared against the model to detect trends or deviations from normal. Depending on the severity of the deviation and trending pattern, Z Operations Analytics will generate an event to alert IT Operations of the anomaly potentially preventing an operational issue if corrective action is taken early. The alert can be consumed by event management systems or correlated with events from the rest of your hybrid cloud with integrated with IBM Cloud Pak for Watson AIOps. The image blow shows an example of real time data (the black line) compared against the model with the lightest blue representing the normal KPI values.
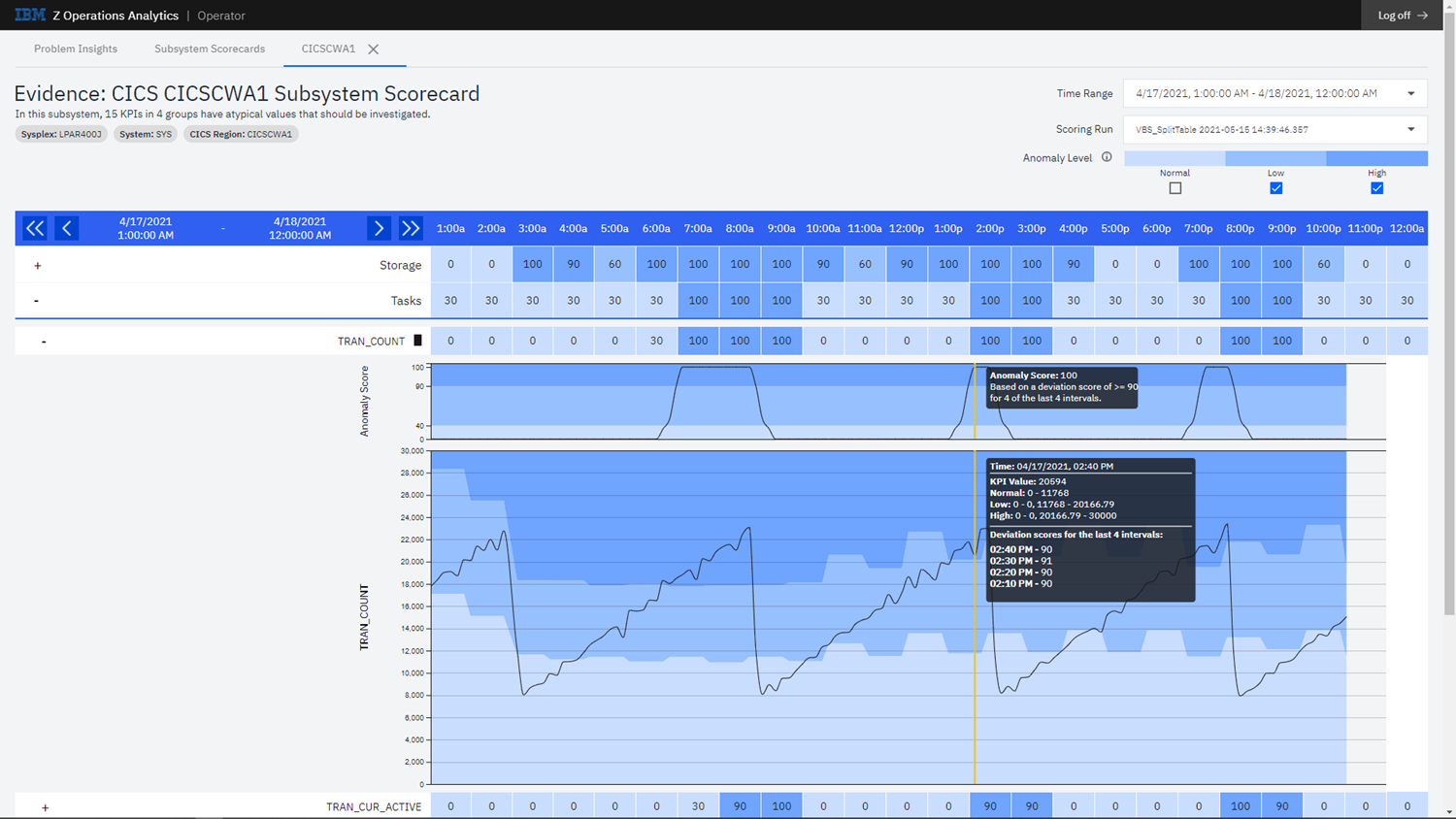
If you would like to get a deeper look into this capability, I recently hosted a webinar where I went into detail on how IBM is leveraging machine learning on IBM Z to detect operational anomalies in order to help our customers avoid costly incidents. The webinar is free to attend and you can register / watch the replay here: https://ibm.biz/AIOps-0601
Originally published on the IBM Z AIOps Community Blog
As Senior Product Manager for the IBM Z AI Ops portfolio, Daniel is responsible for providing business and market direction for IBM solutions that adopt machine learning and AI to help customers avoid critical system outages